In recent years, the fields of Artificial Intelligence (AI) and Machine Learning (ML) have made remarkable progress in the realm of Natural Language Processing (NLP). NLP involves the interaction between computers and human language, enabling machines to understand, interpret, and generate human language. In this article, we explore the intersection of AI and ML in NLP, highlighting the advancements, applications, and exciting possibilities that emerge from this synergy.
Understanding and Interpreting Language
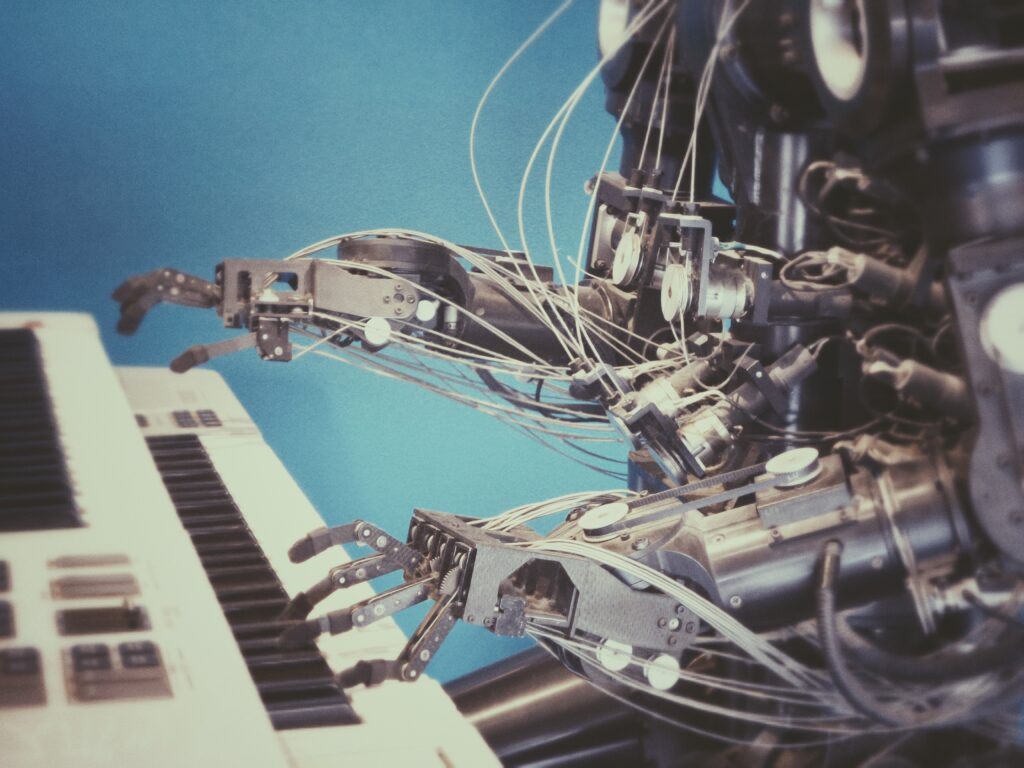
AI and ML algorithms play a pivotal role in understanding and interpreting language. These algorithms are trained on vast amounts of textual data, enabling machines to extract meaning, identify sentiment, and comprehend complex linguistic structures. Techniques such as Natural Language Understanding (NLU) and Named Entity Recognition (NER) utilize ML models to categorize and extract relevant information from text, empowering machines to grasp the nuances of human language.
Language Generation and Conversational AI
AI and ML have revolutionized language generation, enabling machines to produce human-like text. Generative models, such as Recurrent Neural Networks (RNNs) and Transformers, leverage large-scale language models trained on massive datasets to generate coherent and contextually relevant text. Conversational AI, powered by AI and ML, allows machines to engage in human-like interactions, respond to user queries, and hold meaningful conversations. Virtual assistants, chatbots, and voice assistants are some examples of AI-driven conversational agents that rely on NLP techniques.
Sentiment Analysis and Opinion Mining
AI and ML algorithms have significantly advanced sentiment analysis and opinion mining. By analyzing textual data, these algorithms can determine the sentiment expressed in a piece of text, whether it is positive, negative, or neutral. Sentiment analysis finds applications in social media monitoring, customer feedback analysis, brand reputation management, and more. ML algorithms learn from labeled data to classify sentiments accurately, making it possible to gauge public opinion at scale.
Machine Translation and Language Localization
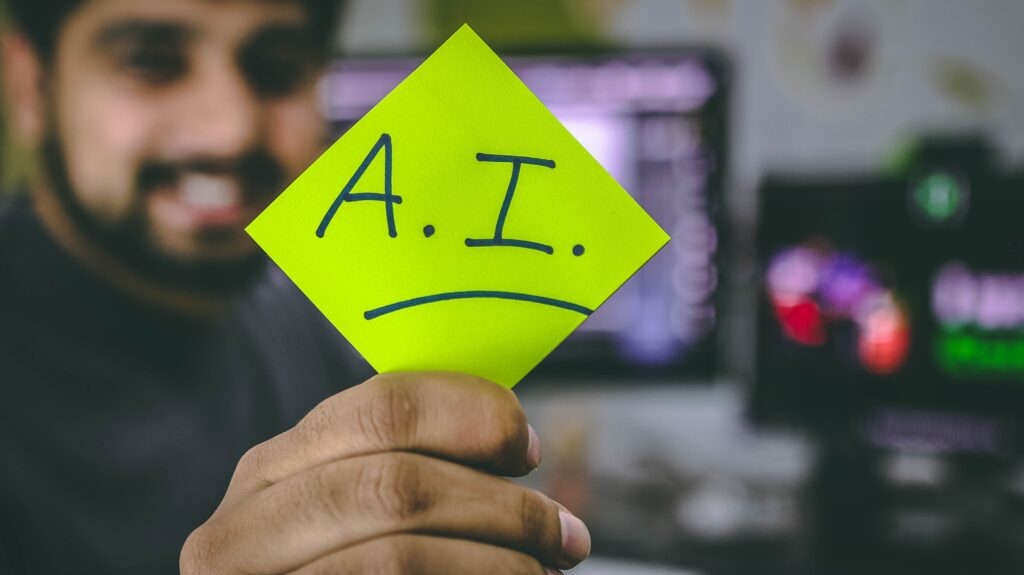
Machine Translation (MT) has witnessed notable advancements due to AI and ML techniques. Neural Machine Translation (NMT), an ML-based approach, has significantly improved translation quality. NMT models learn to map source language to target language by training on extensive parallel corpora. Additionally, AI and ML algorithms aid in language localization by adapting translated content to the cultural and linguistic nuances of the target audience. This ensures that translated text appears natural and contextually appropriate to the local audience.
Semantic Search and Information Retrieval
AI and ML algorithms enhance semantic search and information retrieval systems. By understanding the context and intent behind user queries, these algorithms deliver more relevant search results. ML models, such as deep learning-based ranking algorithms, learn from user interactions and feedback to continuously improve search relevance. Additionally, NLP techniques, such as Named Entity Recognition and Relationship Extraction, aid in extracting structured information from unstructured text, facilitating more precise information retrieval.
Challenges and Future Directions
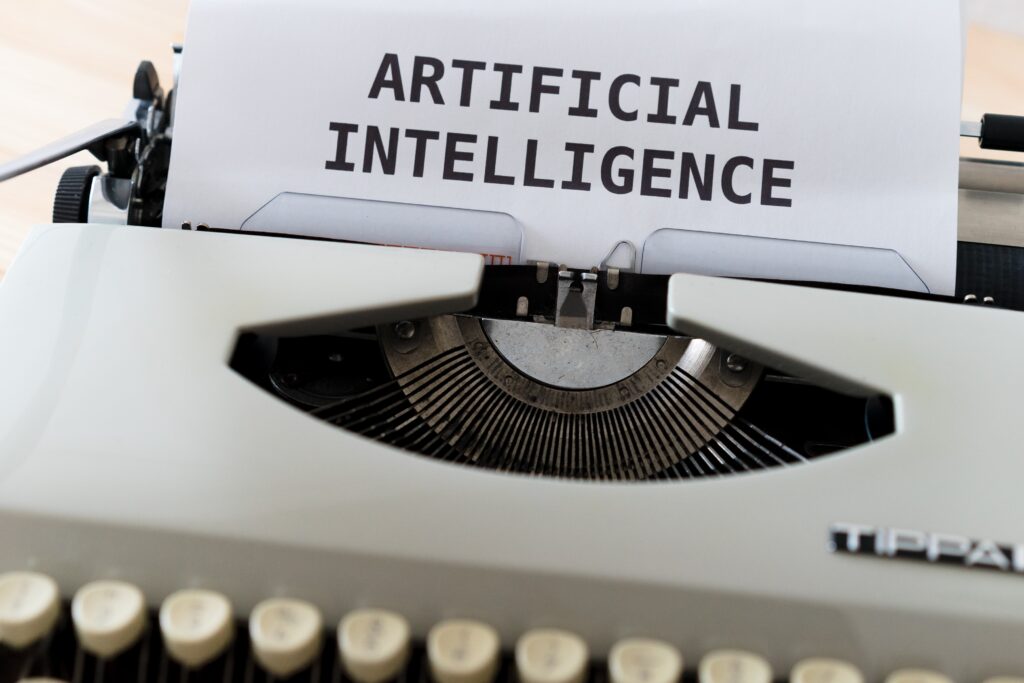
While the collaboration between AI, ML, and NLP has yielded significant advancements, challenges persist. Contextual understanding, ambiguity resolution, and handling complex linguistic phenomena remain ongoing research areas. Additionally, ethical considerations, such as bias in language models and privacy concerns, require attention to ensure responsible and inclusive NLP practices.
The future holds promising possibilities for AI and ML in NLP. Continual advancements in deep learning, reinforcement learning, and unsupervised learning are expected to unlock new frontiers in language understanding and generation. Multimodal NLP, which combines text with other modalities such as images and videos, is an emerging area with exciting potential. Moreover, research in explainable AI and transparent ML models will contribute to building trust and understanding in NLP systems.
Conclusion
The intersection of AI and ML in Natural Language Processing has ushered in a new era of language understanding and generation. From sentiment analysis and language translation to conversational AI and semantic search, AI and ML algorithms have revolutionized how machines comprehend, interact with, and generate human language. As research and innovation continue to push the boundaries, NLP powered by AI and ML holds tremendous potential to transform industries, improve user experiences, and bring us closer to a world where machines truly understand and communicate in our language.
FAQs
How do AI and ML algorithms contribute to natural language understanding?
Answer: AI and ML algorithms analyze vast amounts of textual data to extract meaning, identify sentiment, and comprehend complex linguistic structures. By training on extensive datasets, these algorithms learn to understand and interpret human language.
What are some practical applications of AI and ML in natural language processing?
Answer: AI and ML techniques find applications in various areas of NLP, including language generation, conversational AI, sentiment analysis, machine translation, semantic search, and information retrieval. They enable machines to generate human-like text, hold meaningful conversations, analyze sentiments, translate languages, and retrieve relevant information.
How do AI and ML algorithms improve machine translation quality?
Answer: AI and ML techniques, such as Neural Machine Translation (NMT), enhance machine translation quality. NMT models learn to map source language to target language by training on large parallel corpora. This enables machines to produce more accurate and contextually appropriate translations.
What are the challenges in natural language processing with AI and ML?
Answer: Challenges in NLP with AI and ML include resolving contextual understanding, handling ambiguity, and dealing with complex linguistic phenomena. Ethical considerations related to bias in language models and privacy concerns also need to be addressed to ensure responsible and inclusive NLP practices.
What are the future directions for AI and ML in natural language processing?
Answer: The future of AI and ML in NLP holds exciting possibilities. Advancements in deep learning, reinforcement learning, and unsupervised learning are expected to unlock new frontiers in language understanding and generation. Multimodal NLP, combining text with other modalities, and research in explainable AI and transparent ML models are areas with significant potential for further development.