As technology continues to advance, the threat landscape in cyberspace becomes increasingly complex and sophisticated. Cybersecurity professionals face the daunting task of protecting sensitive data and systems from evolving threats. In this battle against cybercrime, Artificial Intelligence (AI) and Machine Learning (ML) algorithms have emerged as powerful allies. In this article, we delve into the role of AI and ML in enhancing cybersecurity, exploring their capabilities, benefits, and potential challenges.
Identifying and Detecting Threats

One of the primary applications of AI and ML in cybersecurity is threat detection. Traditional signature-based detection methods are limited in their ability to keep up with rapidly evolving threats. AI and ML algorithms, on the other hand, can analyze vast amounts of data, identify patterns, and detect anomalies that may indicate a potential cyberattack. By continuously learning from new data, these algorithms can adapt to emerging threats and detect previously unknown attacks with greater accuracy.
Behavioral Analysis and Anomaly Detection
AI and ML algorithms can analyze user and system behavior to identify deviations from normal patterns. By establishing baseline behavior profiles, these algorithms can detect anomalous activities that may indicate unauthorized access or suspicious behavior. Behavioral analysis enables early detection of insider threats, credential misuse, and other sophisticated attack techniques that may go unnoticed using traditional security measures.
Proactive Threat Hunting and Response
AI and ML algorithms enable proactive threat hunting, empowering cybersecurity teams to identify potential threats before they cause significant damage. By leveraging advanced analytics and predictive modeling, these algorithms can identify patterns and indicators of compromise that may indicate an ongoing or imminent attack. This allows security professionals to take proactive measures to mitigate risks and respond swiftly to emerging threats.
Automated Incident Response and Remediation
AI and ML can automate incident response processes, allowing for faster and more efficient remediation. ML algorithms can analyze historical incident data, identify common patterns, and provide recommendations for incident handling and recovery. Automated incident response systems can also leverage AI algorithms to autonomously investigate and contain threats, minimizing the time and effort required by human responders.
Adaptive Security and Threat Intelligence
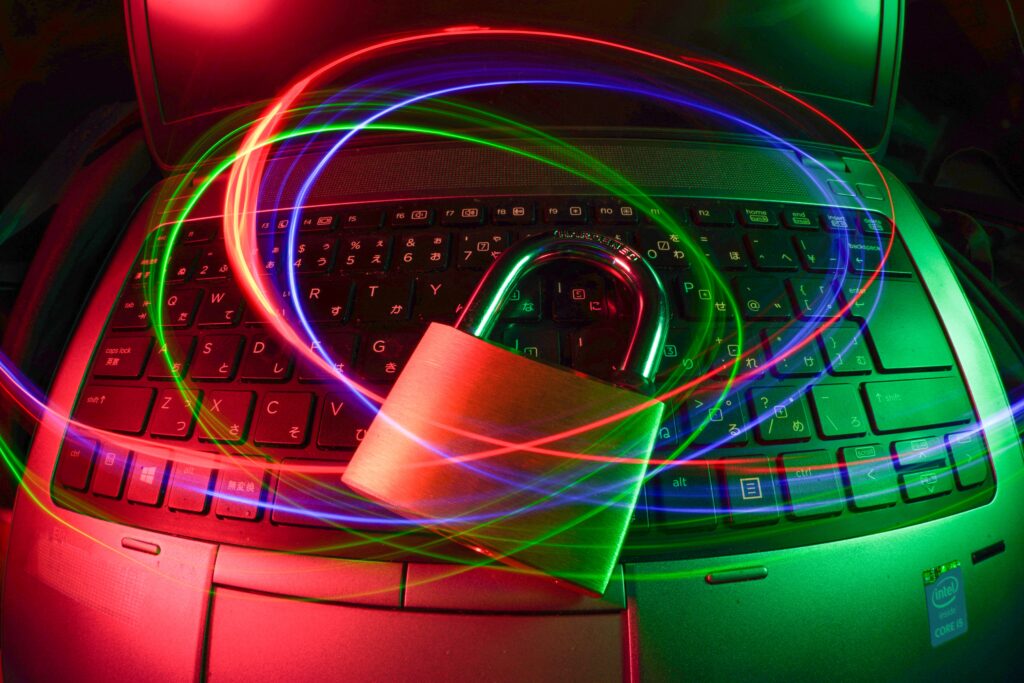
AI and ML algorithms enable adaptive security measures that can continuously learn and adapt to new threats and attack techniques. These algorithms can analyze vast amounts of threat intelligence data, including malware signatures, network traffic patterns, and vulnerability information. By automatically updating and refining their defenses, organizations can stay ahead of evolving threats, reducing the window of vulnerability.
Challenges and Limitations
While AI and ML algorithms offer immense potential for enhancing cybersecurity, they also come with challenges and limitations. Adversarial attacks, where malicious actors attempt to deceive AI algorithms, pose a significant threat. Adversarial machine learning techniques must be developed to enhance the robustness of AI-based security systems. Additionally, ensuring the privacy and security of sensitive data used for training ML models is crucial to prevent unauthorized access or misuse.
Ethical considerations also come into play, such as using AI algorithms responsibly, avoiding bias in decision-making, and addressing potential privacy concerns. Striking a balance between the automation provided by AI and ML and the human oversight and decision-making is essential to maintain effective cybersecurity practices.
Conclusion
Artificial Intelligence and Machine Learning algorithms have revolutionized the field of cybersecurity, providing advanced capabilities for threat detection, anomaly detection, incident response, and adaptive security. By harnessing the power of AI and ML, organizations can enhance their cybersecurity posture, detect and respond to threats more effectively, and stay ahead of evolving cybercriminal tactics. However, it is crucial to address the challenges and ethical considerations associated with these technologies to ensure responsible and secure use. With continued research and collaboration between cybersecurity experts and AI developers, the integration of AI and ML into cybersecurity practices will pave the way for a safer digital future.
FAQs
How do AI and ML algorithms help in detecting sophisticated cyber threats?
Answer: AI and ML algorithms analyze large volumes of data to identify patterns and anomalies that may indicate a potential cyberattack. By continuously learning from new data, these algorithms can adapt to emerging threats and detect previously unknown attacks with greater accuracy.
What is the role of behavioral analysis in cybersecurity?
Answer: Behavioral analysis involves analyzing user and system behavior to identify deviations from normal patterns. AI and ML algorithms establish baseline behavior profiles and can detect anomalous activities that may indicate unauthorized access or suspicious behavior, enabling early detection of insider threats and other sophisticated attack techniques.
How can AI and ML assist in incident response and remediation?
Answer: AI and ML algorithms automate incident response processes by analyzing historical incident data, identifying common patterns, and providing recommendations for incident handling and recovery. Automated incident response systems leverage AI algorithms to autonomously investigate and contain threats, resulting in faster and more efficient remediation.
How do AI and ML algorithms contribute to adaptive security measures?
Answer: AI and ML algorithms enable adaptive security measures by continuously learning and adapting to new threats and attack techniques. They can analyze threat intelligence data, such as malware signatures and network traffic patterns, to automatically update and refine defenses, keeping organizations ahead of evolving threats.
What are the challenges associated with using AI and ML in cybersecurity?
Answer: Adversarial attacks, where malicious actors attempt to deceive AI algorithms, pose a significant challenge. Ensuring the privacy and security of sensitive data used for training ML models is also crucial. Ethical considerations, such as responsible AI use, avoiding bias, and addressing privacy concerns, need to be addressed for the responsible integration of AI and ML in cybersecurity practices.