The Internet of Things (IoT) has ushered in an era of unprecedented data generation, creating massive opportunities and challenges for data analytics and big data. With billions of connected devices producing a constant stream of valuable information, organizations can harness IoT data to gain actionable insights and make data-driven decisions. In this article, we will explore the significant impact of IoT on data analytics and big data, examining how this interconnected ecosystem transforms data collection, storage, processing, and analysis.
IoT Data: Volume, Velocity, and Variety
Data Volume:
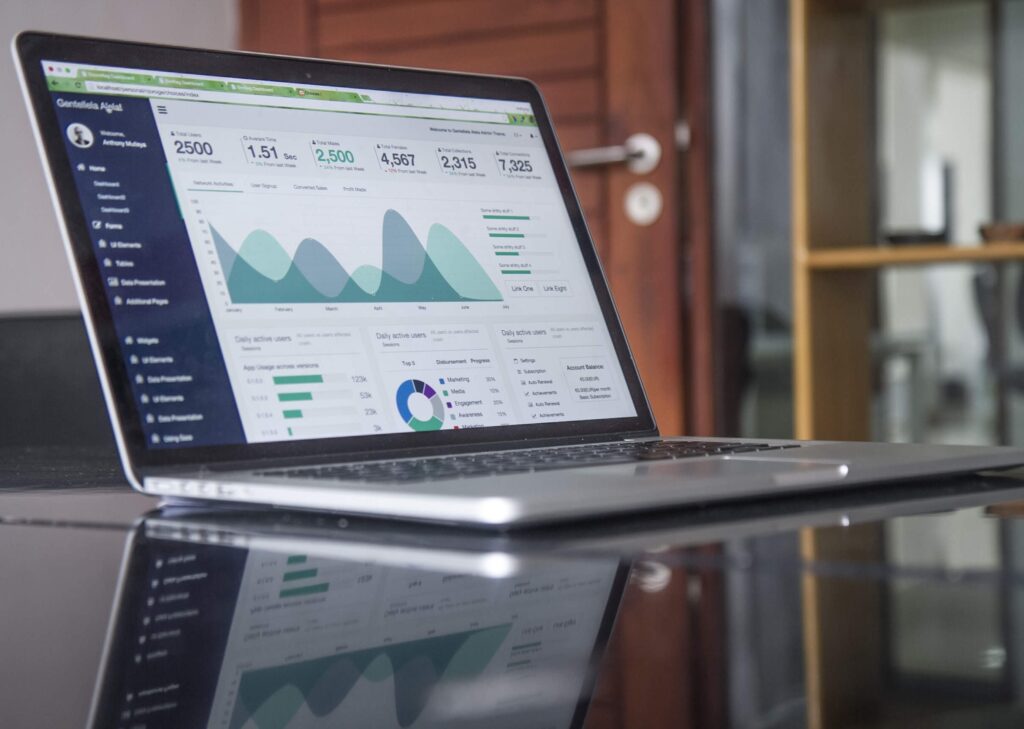
IoT devices generate an enormous volume of data. From sensors embedded in industrial machinery to wearables and smart devices, each connected device continuously produces streams of data, resulting in exponentially larger datasets. This abundance of data presents opportunities for deeper analysis and more accurate insights.
Data Velocity:
The real-time nature of IoT data sets it apart from traditional data sources. IoT devices transmit data continuously and rapidly, often requiring real-time or near-real-time analysis. The high velocity of IoT data necessitates efficient data processing and analysis methods to extract insights in a timely manner.
Data Variety:
IoT data comes in various formats and types, including structured, unstructured, and semi-structured data. It includes sensor readings, images, videos, geospatial data, and more. Managing and analyzing diverse data types require advanced data analytics techniques that can handle and extract insights from this variety of data sources.
Enhanced Data Collection and Connectivity
Granular Data Collection:
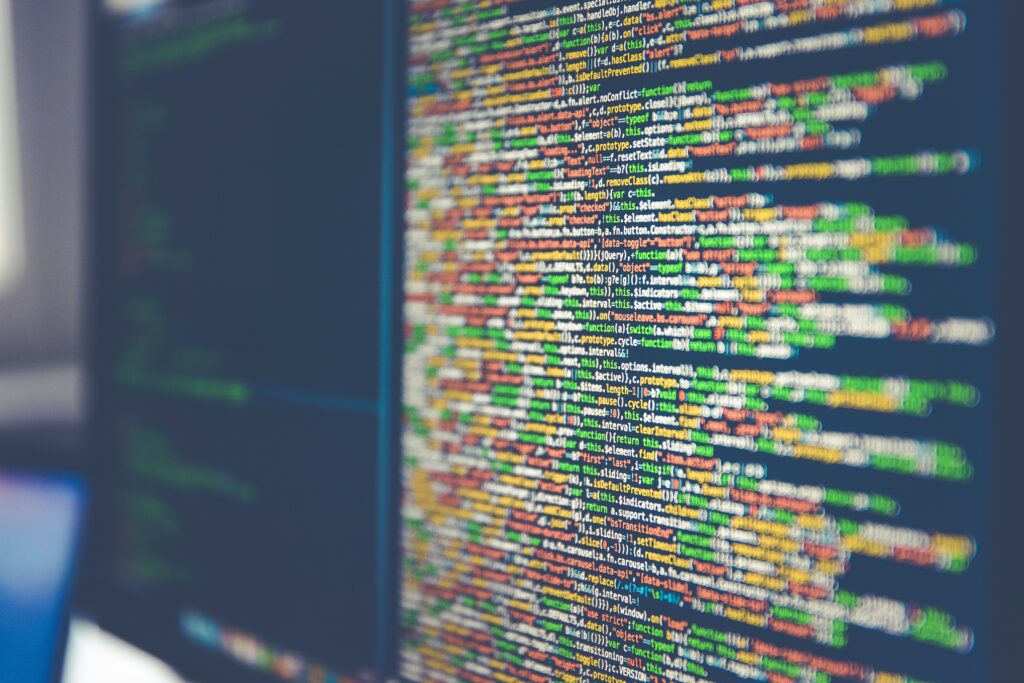
IoT devices collect granular and fine-grained data about the physical world. From environmental conditions to user behavior patterns, IoT sensors capture data at a level of detail previously unattainable. This detailed data provides organizations with a deeper understanding of processes, enabling more accurate analysis and informed decision-making.
Ubiquitous Connectivity:
IoT facilitates seamless connectivity among devices, enabling data to flow across networks without human intervention. This pervasive connectivity ensures that data from various sources can be readily accessible, aggregated, and integrated for comprehensive analysis. The ability to collect data from diverse devices enhances the richness and depth of analytics insights.
Scalable Data Storage and Infrastructure
Cloud Computing:
IoT generates massive amounts of data, requiring scalable and flexible storage solutions. Cloud computing provides the infrastructure needed to store and process IoT data at scale. By leveraging cloud platforms, organizations can store and analyze IoT data without the constraints of on-premises infrastructure, enabling cost-effective scalability and efficient data processing.
Edge Computing:
Edge computing brings data processing closer to the source, reducing latency and alleviating the burden on centralized systems. By analyzing and filtering data at the edge of the network, organizations can extract valuable insights in real time, enabling immediate action and reducing dependence on cloud infrastructure for data processing.
Advanced Analytics and Real-time Insights
Predictive Analytics:
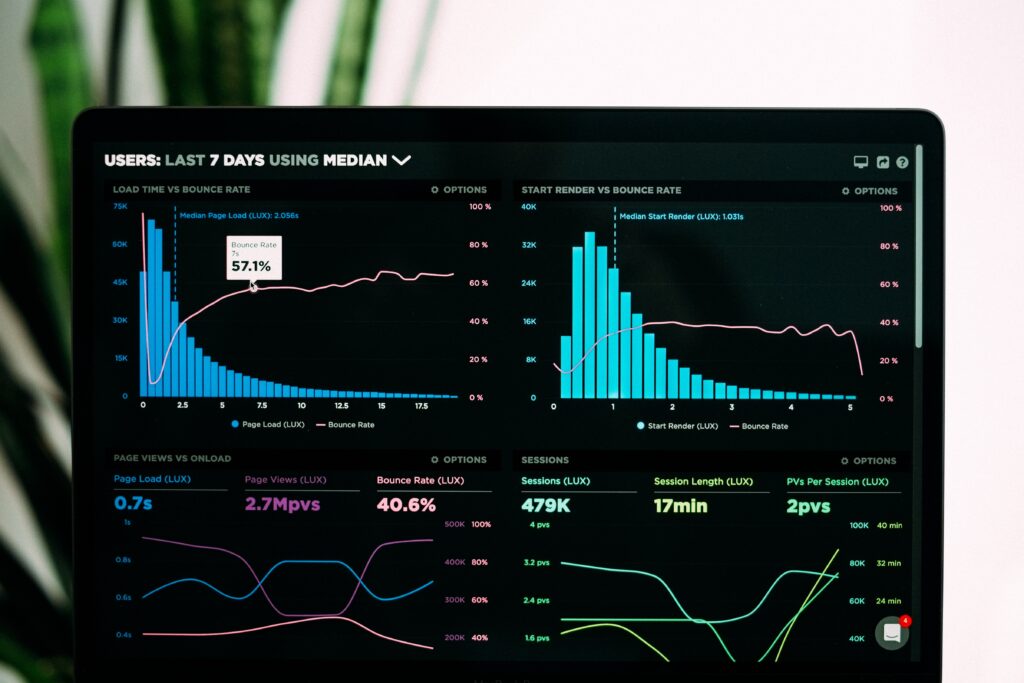
IoT data empowers organizations to leverage predictive analytics to anticipate events and make proactive decisions. By analyzing historical IoT data, organizations can identify patterns, detect anomalies, and predict future outcomes. This predictive capability enables proactive maintenance, improved operational efficiency, and enhanced customer experiences.
Machine Learning and AI:
IoT data provides a rich source for training machine learning models and developing artificial intelligence applications. By leveraging IoT data, organizations can build predictive models, automate processes, and develop intelligent applications that learn from real-time data, leading to improved efficiency, accuracy, and innovation.
Security and Privacy Considerations
Data Security:
The sheer volume and sensitivity of IoT data raise significant security concerns. Organizations must implement robust security measures to protect IoT data throughout its lifecycle. This includes secure device authentication, data encryption, access control, and regular security updates to mitigate the risk of data breaches and unauthorized access.
Privacy Protection:
IoT data often contain personal and sensitive information, necessitating adherence to privacy regulations. Organizations must implement privacy-by-design principles, anonymize or pseudonymize data where necessary, and ensure transparency and user consent in data collection and processing activities to maintain privacy standards.
Conclusion
The Internet of Things has revolutionized data analytics and big data, opening up new possibilities for organizations to harness the power of real-time, granular data. IoT’s impact on data analytics spans data collection, storage, processing, and analysis, enabling organizations to derive actionable insights, make data-driven decisions, and drive innovation. However, as the IoT ecosystem continues to expand, organizations must address security and privacy challenges to ensure the responsible and secure utilization of IoT data. By leveraging IoT data effectively, organizations can unlock valuable insights and drive transformative changes across industries.
FAQs
What is the relationship between IoT and big data analytics?
Answer: IoT and big data analytics are closely intertwined. IoT generates massive volumes of data, and big data analytics provides the tools and techniques to process and derive insights from this data. IoT feeds into big data analytics by providing real-time, granular data that can be analyzed to gain actionable insights and drive informed decision-making.
How does IoT impact data storage requirements?
Answer: IoT generates vast amounts of data, which necessitates scalable and flexible storage solutions. Cloud computing plays a significant role in meeting the storage needs of IoT data, allowing organizations to store and process data in a cost-effective and scalable manner. Edge computing also reduces the burden on centralized systems by processing data closer to the source, enabling real-time analysis and immediate action.
What are the benefits of real-time data analytics in the context of IoT?
Answer: Real-time data analytics in IoT enables organizations to respond quickly to events, detect anomalies, and make proactive decisions. By analyzing IoT data in real-time, organizations can gain insights that drive operational efficiency, predictive maintenance, and improved customer experiences. Real-time analytics empowers organizations to take immediate actions based on up-to-date information.
How does IoT data contribute to predictive analytics?
Answer: IoT data plays a crucial role in predictive analytics by providing historical data for pattern recognition and trend analysis. By analyzing past IoT data, organizations can identify patterns, detect anomalies, and develop predictive models. These models enable organizations to anticipate future events, make proactive decisions, and optimize processes for improved efficiency and performance.
What security and privacy considerations are important when working with IoT data?
Answer: Security and privacy are paramount when dealing with IoT data. Organizations must implement robust security measures, including secure device authentication, data encryption, and access control, to protect IoT data from breaches and unauthorized access. Privacy protection is also essential, requiring adherence to privacy regulations, transparency in data collection, and user consent to ensure responsible and ethical use of IoT data.