Machine learning has emerged as a powerful tool in data engineering, enabling advanced analytics and predictive insights. This article explores the integration of machine learning in data engineering, its impact on predictive analytics, and the distinct competitive advantages it offers. Additionally, we delve into how machine learning accelerates analytics processes and reduces costs, making it a valuable asset for organizations.
I. A New Dimension in Predictive Analytics:
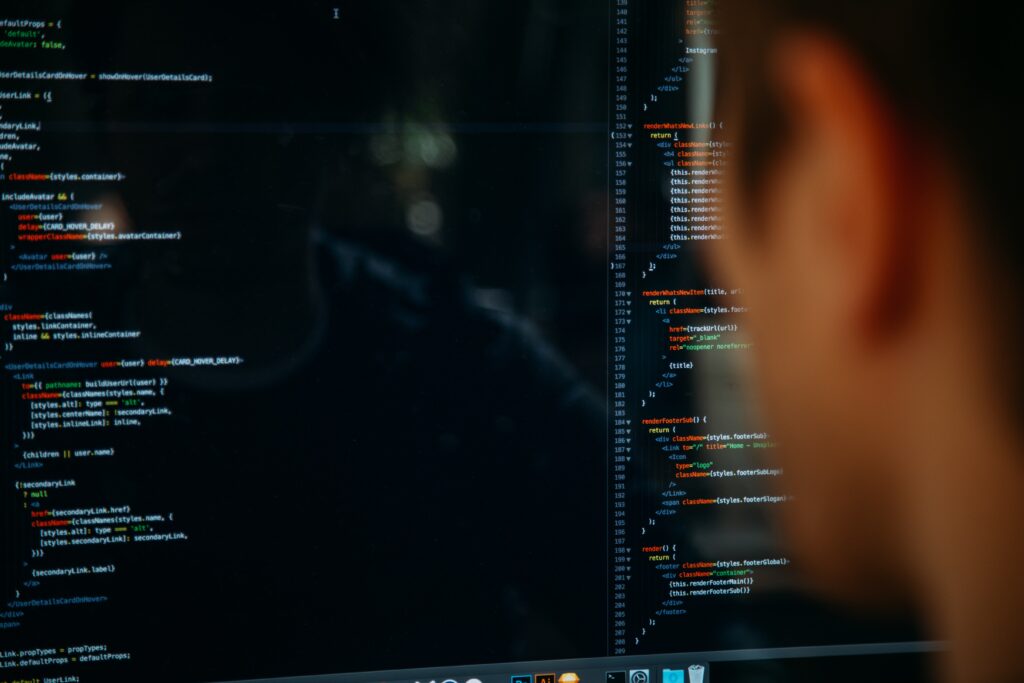
Machine learning brings a new dimension to predictive analytics by leveraging algorithms and statistical models to identify patterns, make predictions, and generate insights from vast amounts of data. It enables data engineers to extract meaningful information and uncover hidden relationships that traditional analytics approaches may not capture.
Artificial Intelligence (AI) provides a significant boost to data engineering, revolutionizing the way organizations process, manage, and derive insights from their data. AI techniques, such as machine learning and deep learning, augment data engineering capabilities by automating complex tasks, improving data quality, and uncovering hidden patterns in large datasets.
AI-driven algorithms enable data engineers to develop more sophisticated data pipelines, enhance data integration and transformation processes, and accelerate data processing and analysis. Furthermore, AI-powered data engineering solutions enhance efficiency, scalability, and accuracy, enabling organizations to harness the full potential of their data and drive data-centric innovation. With AI, data engineering becomes more intelligent, efficient, and capable of delivering valuable insights for informed decision-making.
II. Predictive Analytics Is Everywhere:
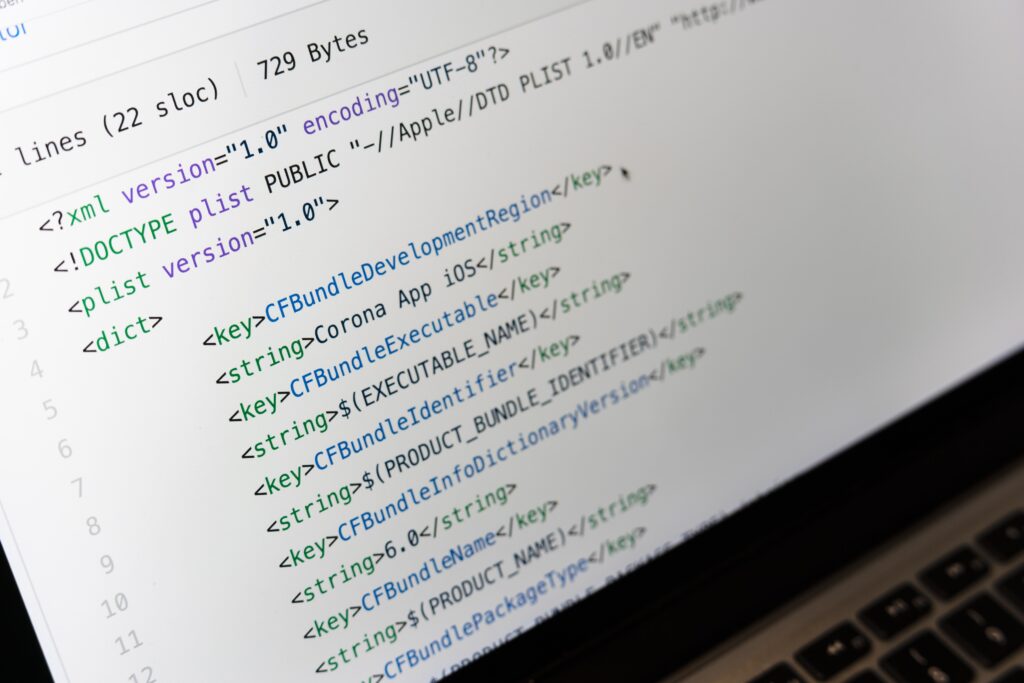
Machine learning has found applications in various domains, including finance, healthcare, marketing, cybersecurity, and more. It empowers organizations to make data-driven predictions, such as customer behavior, fraud detection, disease diagnosis, demand forecasting, and anomaly detection.
III. Distinct Competitive Advantage:
Integrating machine learning into data engineering provides organizations with a distinct competitive advantage. By leveraging predictive insights, businesses can make informed decisions, optimize processes, personalize customer experiences, detect emerging trends, and gain a deeper understanding of their operations and target markets.
IV. Faster and Less Expensive:
Machine learning algorithms automate the analysis of large datasets, significantly reducing the time and resources required for complex analytics tasks. By leveraging machine learning in data engineering, organizations can accelerate the speed at which predictive models are developed and deployed, leading to faster insights and more agile decision-making processes.
V. Conclusion:
Machine learning has revolutionized the field of data engineering, enabling advanced analytics and predictive insights. By leveraging machine learning algorithms, organizations can unlock valuable patterns and trends within their data, gaining a competitive edge in the market. Additionally, machine learning accelerates analytics processes, providing faster and more cost-effective solutions. Embracing machine learning in data engineering is a strategic move for organizations looking to harness the power of predictive analytics and drive data-centric innovation in their operations.
FAQs
What is the role of machine learning in data engineering?
Machine learning plays a crucial role in data engineering by enabling advanced analytics and predictive insights. It leverages algorithms and statistical models to analyze large datasets, identify patterns, and make predictions, empowering organizations to extract meaningful information and gain a competitive edge.
How does machine learning enhance predictive analytics?
Machine learning enhances predictive analytics by automating the analysis of data, uncovering hidden relationships, and generating accurate predictions. It enables organizations to make data-driven decisions, optimize processes, personalize customer experiences, detect anomalies, and gain deeper insights into their operations.
In which industries can machine learning be applied for predictive analytics?
Machine learning can be applied in various industries, including finance, healthcare, marketing, cybersecurity, and more. It offers predictive capabilities for tasks such as customer behavior analysis, fraud detection, disease diagnosis, demand forecasting, and anomaly detection.
What competitive advantages does machine learning offer in data engineering?
By integrating machine learning into data engineering, organizations gain a distinct competitive advantage. They can make informed decisions based on predictive insights, optimize processes for improved efficiency, deliver personalized experiences to customers, detect emerging trends, and gain a deeper understanding of their operations and target markets.
How does machine learning in data engineering accelerate analytics processes?
Machine learning algorithms automate the analysis of large datasets, reducing the time and resources required for complex analytics tasks. By leveraging machine learning in data engineering, organizations can develop and deploy predictive models faster, leading to quicker insights and more agile decision-making processes. This acceleration allows organizations to respond swiftly to changing market conditions and gain a competitive edge.