Autonomous vehicles have emerged as one of the most exciting and transformative applications of artificial intelligence (AI) and machine learning (ML) technologies. With the potential to revolutionize transportation, these self-driving vehicles rely on advanced AI and ML algorithms to perceive their surroundings, make informed decisions, and navigate safely on the roads. In this article, we explore the crucial role of AI and ML in autonomous vehicles, their capabilities, and the challenges they face on the path to widespread adoption.
Perception and Sensor Fusion
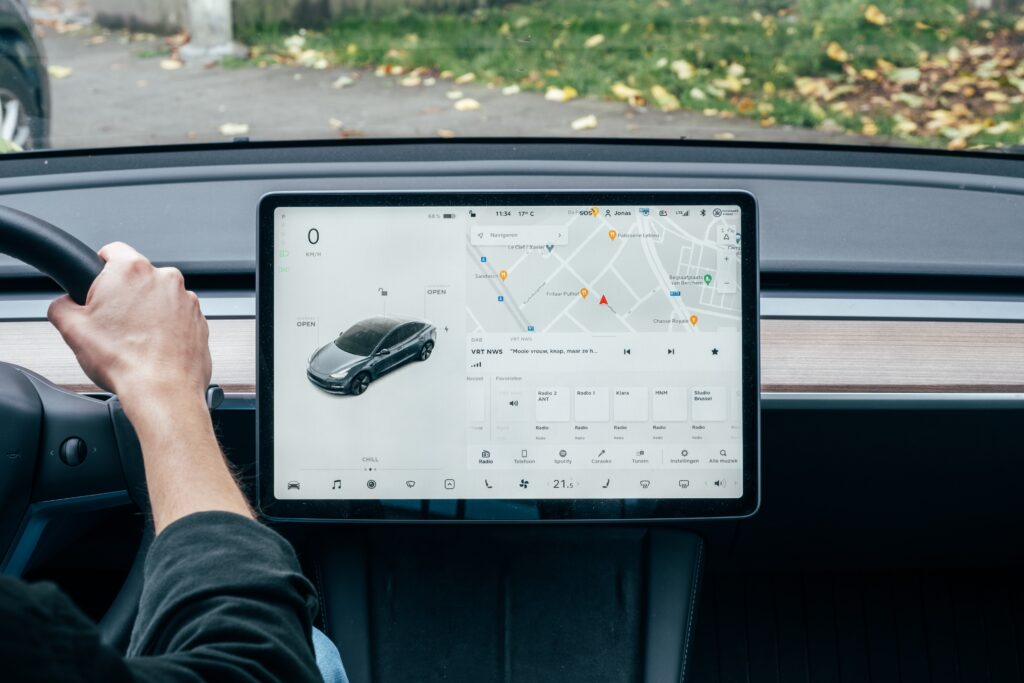
A fundamental aspect of autonomous vehicles is their ability to perceive and understand their surroundings. AI and ML algorithms analyze data from a variety of sensors, including cameras, radar, lidar, and ultrasonic sensors, to create a comprehensive and accurate view of the environment. Through sensor fusion techniques, which combine data from multiple sensors, the vehicle can detect and track objects, identify traffic signs, recognize pedestrians, and interpret complex traffic scenarios.
Decision-Making and Planning
AI and ML play a vital role in the decision-making and planning process of autonomous vehicles. These technologies enable the vehicle to analyze and interpret the perceived data to make informed decisions in real time. ML algorithms can learn from vast amounts of data, including historical driving patterns and experiences, to improve decision-making capabilities.
By incorporating reinforcement learning techniques, autonomous vehicles can continually adapt and optimize their actions based on feedback from the environment. This adaptive decision-making allows the vehicle to handle complex scenarios, such as merging lanes, changing traffic conditions, and unexpected obstacles.
Safety and Risk Assessment
Ensuring the safety of autonomous vehicles and their passengers is of paramount importance. AI and ML algorithms play a significant role in assessing and mitigating potential risks on the road. Through advanced algorithms, autonomous vehicles can detect and predict the behavior of other vehicles, assess potential collision risks, and take proactive measures to avoid accidents.
ML techniques can also help vehicles learn from near-miss situations and unusual events, improving their ability to handle challenging situations effectively. This continuous learning and risk assessment process contribute to the overall safety and reliability of autonomous vehicles.
Mapping and Localization
Accurate mapping and localization are critical components for autonomous vehicles to navigate successfully. AI and ML algorithms help create and update high-definition maps, incorporating real-time data to ensure precise positioning and environmental understanding.
Simultaneous Localization and Mapping (SLAM) techniques enable autonomous vehicles to build and maintain a map of their surroundings while simultaneously tracking their own position within that map. AI algorithms aid in optimizing and refining the SLAM process, allowing vehicles to navigate with accuracy, even in complex urban environments or areas with limited GPS coverage.
Challenges and Ethical Considerations
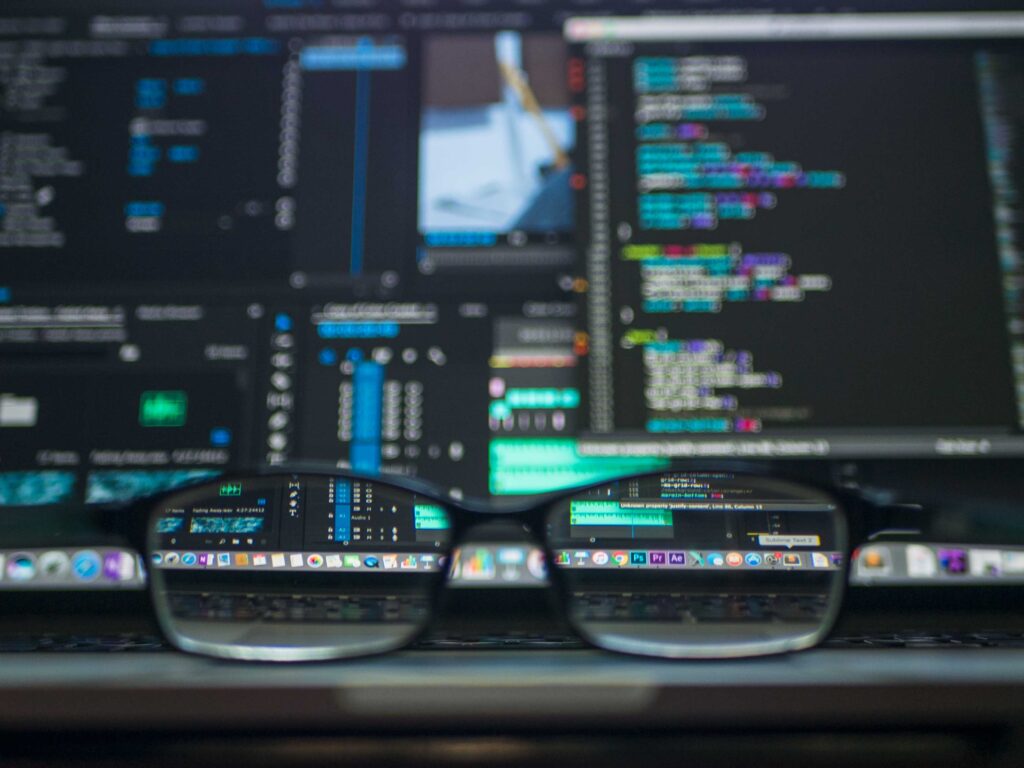
While AI and ML offer immense potential for autonomous vehicles, several challenges and ethical considerations need to be addressed. Safety remains a top concern, as these vehicles must operate flawlessly to avoid accidents. Ensuring the security and robustness of AI systems against potential cyber threats is also crucial.
Ethical dilemmas arise when determining how autonomous vehicles should prioritize decisions in potential accident scenarios. Striking the right balance between protecting occupants, pedestrians, and other vehicles poses complex ethical and moral challenges that require careful consideration and societal consensus.
Regulatory and Legal Frameworks
The widespread adoption of autonomous vehicles necessitates the development of robust regulatory and legal frameworks. Governments and policymakers must establish guidelines and standards for testing, deployment, and operation. These frameworks should address safety requirements, liability allocation, data privacy, and cybersecurity to ensure the responsible and safe integration of autonomous vehicles into existing transportation systems.
Conclusion
The role of AI and ML in autonomous vehicles is revolutionizing transportation as we know it. By enabling perception, decision-making, planning, and risk assessment, these technologies are making self-driving vehicles a reality. While significant progress has been made, challenges such as safety, ethics, and regulatory frameworks must be carefully addressed to fully realize the potential of autonomous vehicles. As technology continues to advance, autonomous vehicles have the potential to enhance road safety, reduce congestion, and transform the way we travel.
FAQs
How do AI and ML enable autonomous vehicles to perceive and understand their surroundings?
Answer: AI and ML algorithms analyze data from sensors such as cameras, radar, lidar, and ultrasonic sensors to create a comprehensive view of the environment. Through sensor fusion techniques, the vehicle can detect and track objects, identify traffic signs, recognize pedestrians, and interpret complex traffic scenarios.
How do AI and ML algorithms contribute to the decision-making process of autonomous vehicles?
Answer: AI and ML algorithms analyze the perceived data to make informed decisions in real time. By incorporating reinforcement learning techniques, autonomous vehicles can adapt and optimize their actions based on feedback from the environment, allowing them to handle complex scenarios and unexpected obstacles.
What role do AI and ML play in ensuring the safety of autonomous vehicles?
Answer: AI and ML algorithms help autonomous vehicles assess and mitigate potential risks on the road. By detecting and predicting the behavior of other vehicles, assessing collision risks, and taking proactive measures, autonomous vehicles prioritize safety and avoid accidents. ML techniques also enable vehicles to learn from near-miss situations and unusual events, improving their ability to handle challenging scenarios effectively.
How do AI and ML algorithms contribute to accurate mapping and localization in autonomous vehicles?
Answer: AI and ML algorithms help create and update high-definition maps, incorporating real-time data for precise positioning and environmental understanding. Simultaneous Localization and Mapping (SLAM) techniques allow vehicles to build and maintain maps of their surroundings while tracking their own position within those maps. This ensures accurate navigation, even in complex urban environments or areas with limited GPS coverage.
What are the key challenges and ethical considerations associated with autonomous vehicles and AI/ML technology?
Answer: Safety is a primary concern, and ensuring the security and robustness of AI systems against cyber threats is crucial. Ethical dilemmas arise when determining how autonomous vehicles should prioritize decisions in potential accident scenarios. Striking the right balance between protecting occupants, pedestrians, and other vehicles poses complex ethical and moral challenges. Additionally, developing regulatory and legal frameworks that address safety requirements, liability allocation, data privacy, and cybersecurity is essential for the responsible integration of autonomous vehicles into existing transportation systems.